A CISO’s perspective on how to understand and address AI risk
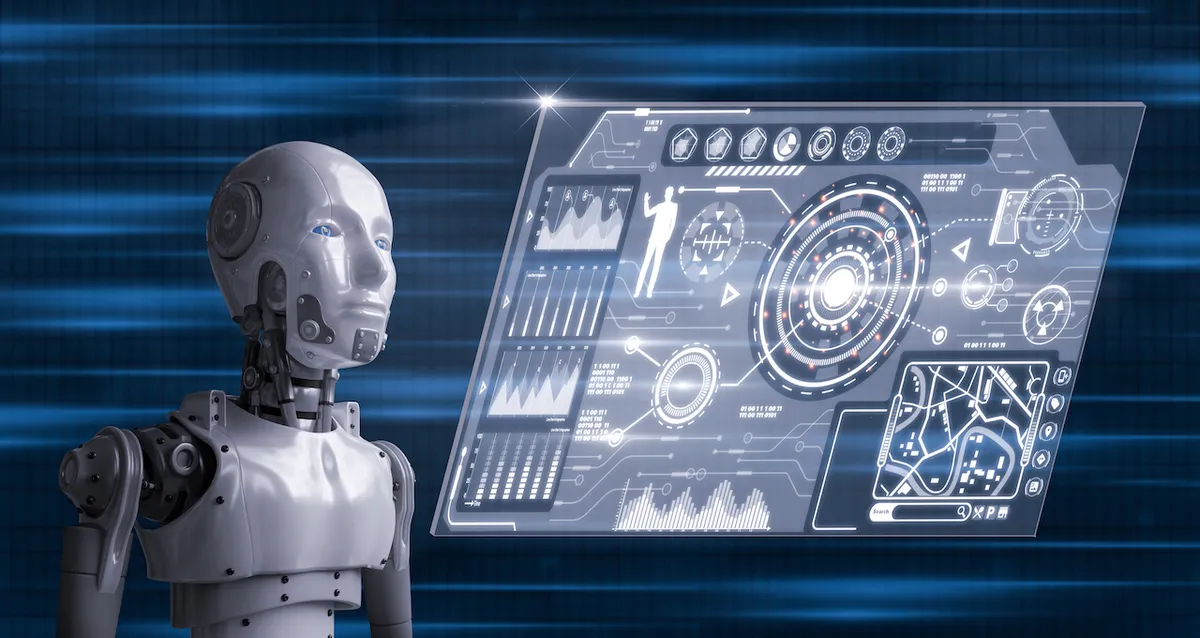
Written by Diana Kelley for SC Media
Artificial intelligence (AI) has quickly reshaped the business world. It’s unleashing changes that extend across sectors, industries, and companies. Already, the technology touches nearly every aspect of business — and its impact continues to grow.
Yet, amid all the possibilities and opportunities, there are also substantial risks related to using AI in the enterprise. These include technical, reputational, regulatory, and operational risks that can result in exploits of supply chain flaws and lead to system downtime, theft of credentials and PII, non-compliance with laws and regulations, and loss of brand trust.
As we increase our dependence on these systems, business and IT leaders — particularly CIOs, CISOs, CTOs, and CDOs on the front lines of technology adoption — must also increase their ability to trust that the outputs of AI are being delivered safely and securely, and ensure that they operate in a way that maintains a strong security posture.
Avoid risky business
Although 76% of companies deploying AI have policies, and procedures in place to manage risk, only 24% of executives believe that the policies are even “somewhat effective,” according to a survey by international law firm Baker McKenzie. Furthermore, the risks of AI are misunderstood, with only a little over 52% believing the risks are somewhat significant.
I’ve categorized four core areas of AI risk:
- Technical: These include technical issues and vulnerabilities in the AI/ML lifecycle and supply chain. For example, a popular open source tool, MLFlow has been vulnerable to local file include/remote file include attacks that could lead to complete system takeover. Research from Synopsys found that more than 80% of analytics, big data, and AI workloads contain OSS assets in their supply chain. A lot of those are ML models downloaded from repositories like Hugging Face. ML models can execute code and get artifacts, and are often run with high privilege. We’d expect a company to scan a PDF in email before opening it, but few companies do any scanning of downloaded models before use, leaving them vulnerable to attacks.
- Operational: As AI and ML evolve and adoption grows, organizations run the risk of becoming overly dependent on these tools — particularly for automated tasks. Without adequate oversight and controls, systems might behave unpredictably, inaccurately or simply fail. The fallout could range from frustrating to catastrophic. An organization might wind up with unanticipated production errors, operationally significant downtime, even accusations of discrimination. It’s vital to ensure that an AI or ML system continues to operate as designed — and intended.
- Regulatory: Government agencies and regulators are ratcheting up their oversight of AI and ML. The EU has developed the EU AI Act, Canada has released the Artificial Intelligence and Data Act, and President Biden issued an executive order on AI. The result? The regulation of AI and its security is coming. Organizations must evaluate how AI and ML fit into regulatory structures and take appropriate steps to mitigate regulatory risk and stay compliant.
- Reputational: Organizations earn a reputation slowly over time, but can lose it in an instant. As enterprises turn to AI for their customer-facing workflows, failures can lead to loss of brand trust.
For example, a financial institution using a rogue robo-advisor could deliver bad or incorrect information to investors — or make an error when managing or recalibrating a stock portfolio. In either scenario, the resulting press or social media attention could significantly undermine customer trust and degrade brand integrity.
Dial down the risks
A best practice approach to reducing risk revolves around these important tenants:
- Build security in with MLSecOps. It’s vital to integrate security into an MLOps Lifecycle, in much the same way that security gets woven into DevOps. This requires security touchpoints — including monitoring and testing — throughout the AI/ML lifecycle, from early development to deployment and ongoing use.
- Foster cross-team collaboration. Encourage collaboration across security, ML teams, and other relevant departments such as human resources and legal. This supports a comprehensive understanding of AI applications along with visibility about the full range of their impacts across the organization.
- Introduce threat modeling. Use threat modeling exercises to identify vulnerabilities in AI/ML systems early on so the team can test thoroughly before use. This practice helps identify risks and offers broader and deeper insight into how AI impacts security as well as the overall business.
- Adopt regular testing and monitoring. Continuously test and monitor AI systems to ensure they operate as designed — and as expected. This includes checking for model drift, biases, and operational effectiveness. In a best practice framework, security and machine learning teams work together to build robust guardrails and controls.
- Stay informed on regulatory changes. Keep an eye on emerging regulations and standards related to AI and ML. Ensure the organization's AI applications are compliant and be prepared for further regulatory frameworks.
- Patch and update. Monitor and patch all tools in the lifecycle, including open source and remove at risk dependencies.
- Focus on zero-trust principles. To limit the blast radius of an attack or failure, implement protective architectural approaches like least privileged access, sandboxing and segmentation, and multi factor authentication.
Make no mistake, AI is here to stay — and it represents an enormous opportunity. Yet, it’s up to business and technology leaders to ensure that the technology remains safe and secure, and vulnerabilities are managed.