Security: The Most Ignored Area of MLOps
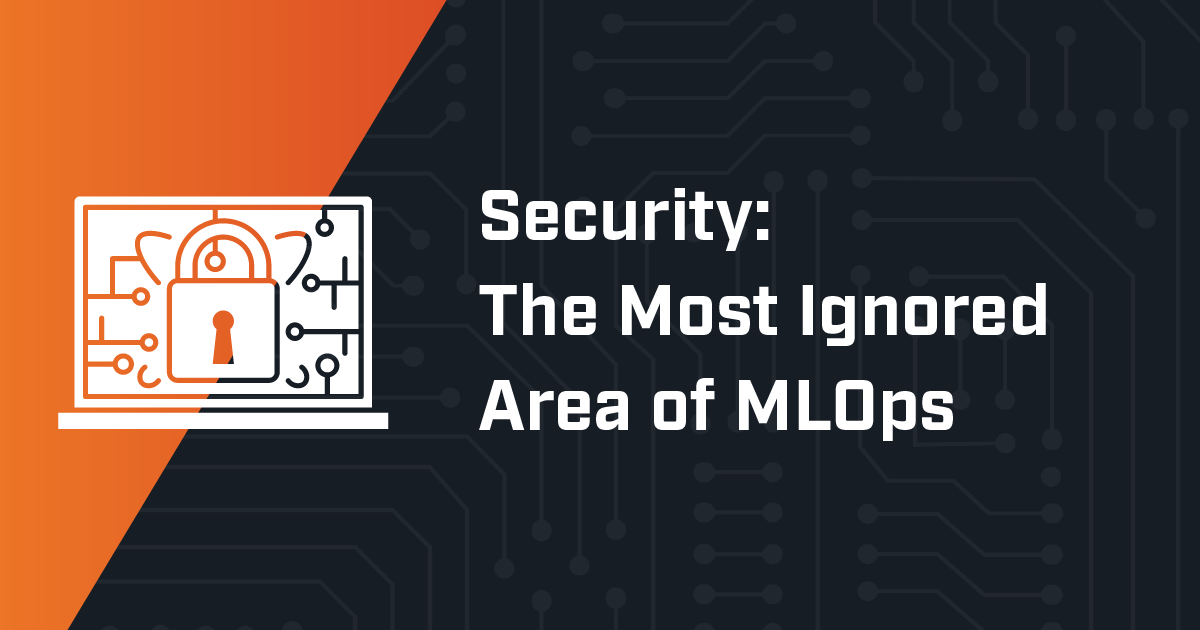
ML pipelines are unique and challenging to secure. Jesus Rodriguez shares his thoughts on the unique attack surfaces ML applications have and how security is treated as an afterthought.
Editorial: Security: The Most Ignored Area of MLOps
Jesus Rodriguez | The Sequence Scope: Security: The Most Ignored Area of MLOps
In the last few years, we have seen remarkable levels of innovations across most areas of the MLOps stack. Model serving, monitoring, interpretability, testing are some areas that have quickly become incredibly fragmented with numerous innovative startups and incumbents launching incredibly compelling offerings. Security seems to be the one area lacking behind in innovation in the ML space. This might seem surprising as, in the traditional DevOps space, security have become an integral part of the lifecycle of applications. In the case of ML, security is often treated as an afterthought or try to be addressed by using traditional stacks which don’t quite adapt to the dynamics of ML applications.
Securing ML pipelines is not only different but quite challenging. The nature and surface of attacks in ML solutions doesn’t share the DNA of traditional applications often involving areas such as data or policy manipulation. This problem is even worse in the era of large foundation models which are currently dominating the ML landscape. If we don’t even understand how a large model makes predictions, how can we protect it?
Just like MLOps was the evolution of DevOps for the ML era, ML security needs a new stack. The ML space needs a new generation of ML-first security platforms. Most of the innovation in ML security has been constrained to research and experimental efforts but that’s starting to change. Last week, ML security Protect.ai came out of stealth mode announcing a new series A and one of the most complete and pragmatic ML-first security stacks ever released. Their initial platform is segmented in two fundamental product. NB Defense is a tool that scans for security vulnerabilities directly in Jupyter networks which incentivizes data scientists to incorporate security since the experimentation phase of an ML solution. AI Radar will be the second product of Protect.ai with a focus on enabling more comprehensive suite for testing and discovering vulnerabilities in ML pipelines.
From networking to cloud computing, every software trend in history have created a parallel cyber security industry. ML needs ML-first security. Protect.ai is a good starting point but we are likely to see security evolve into its own market in the ML space.